I’m Shnay, the Senior Product Manager for Monzo's Customer Support Experience Squad. Our team ensures that customers get the best help and support experience on the app. This involves managing self-serve journeys and overseeing the help tab. I recently wrote ‘a week in the life of a Product Manager at Monzo’ if you want to learn more about what a Product Manager does.
Over the last year we launched 43 experiments, this blog post conveys some of the most interesting things we’ve learned.
Experimentation is a critical part of product development, and the benefits of running experiments are well-known. Experimentation allows product teams to test their assumptions and validate their hypotheses, which ultimately leads to better products and better customer experiences.
Some of the changes we experimented with include:
Creating a Chat Form to help customers get the support they need quicker
Providing Quick Links on the Help Tab to allow customers to self-serve easier
Auto recommending search suggestions for customers in help
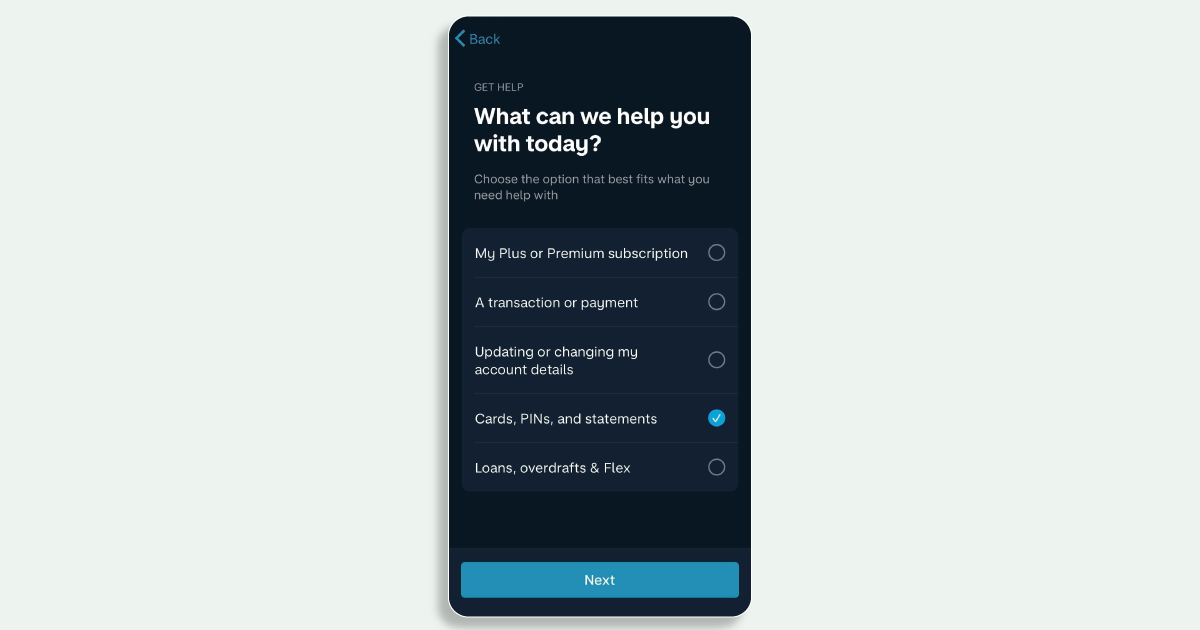
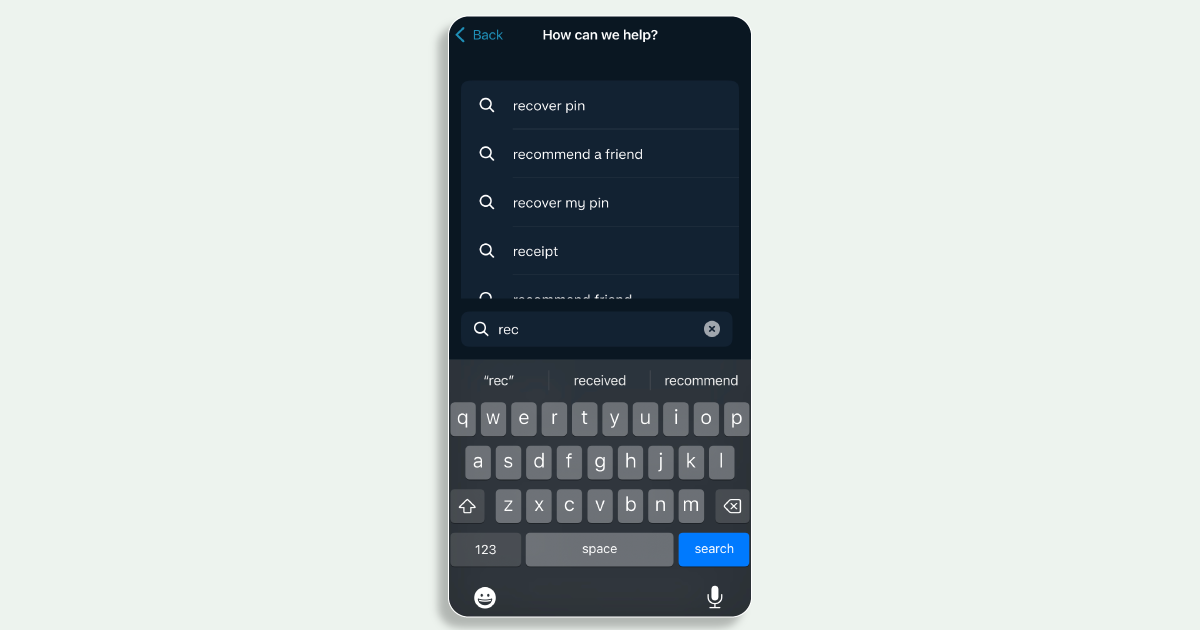
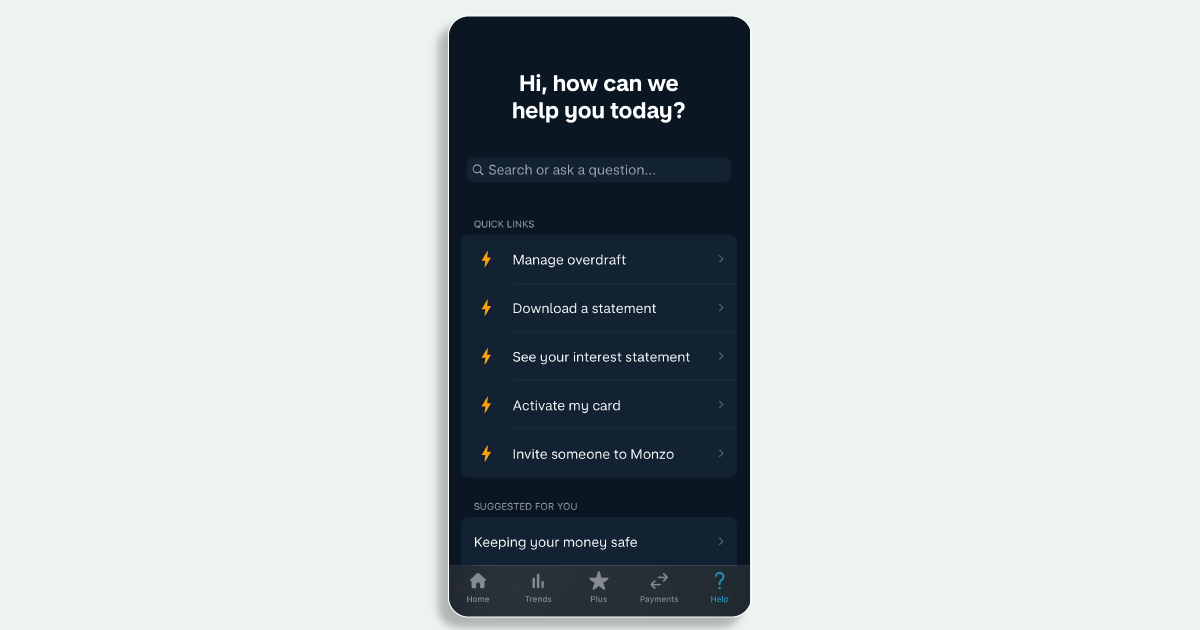
Start with a clear hypothesis: Having a clear hypothesis is imperative because it sets the foundation for the experiment and guides the team's decision-making process. A hypothesis is a statement that proposes a solution to a problem, or a potential outcome for an experiment, which ultimately helps the team learn what does and does not work. It should be specific, measurable, and testable, for example - ‘by providing search suggestions to customers using help search we will improve our customer effort score whilst maintaining the cost per weekly active user’. Here we know the user, business impact and how we will prove or disprove it. Whereas a bad example could be ‘search suggestions will help every customer solve their problem’. Without a clear hypothesis, the team may not understand what they're trying to achieve or how to measure success. A well-defined hypothesis helps the team focus their efforts, isolate variables, and measure the impact of the experiment accurately. It also helps the team avoid making assumptions or relying on guesswork, which can lead to wasted resources and inaccurate results.
Measuring against the right metrics: When conducting experiments, it's essential to measure metrics that are relevant to the experiment's objective and to the overall product vision. Equally it’s important to have guardrail metrics, to make sure your change is safe and avoids any unintended consequences. For example if you are optimising to save costs but want to ensure the customer experience is not being negatively affected then it’s important to measure both in your experiment. As an extreme case, you wouldn’t want to stop answering customers' phone calls because it would save you money.
Make sure your experiment is well isolated: A/B testing is a common method for product teams to experimentally test the effectiveness of changes made to a product. However, to accurately measure the impact of the changes being tested, the experiment must be well-isolated. This means two things:
The control and enabled groups do not mix or overlap
Those that are assigned to the experiment also have a change in experience
In some cases, the changes being tested may depend on user actions, such as clicking a button. If you start measuring this when a customer lands on the page, as opposed to when they tap the button, it could lead to dilution of the experiment, making it harder to reach statistical significance. Reaching statistical significance proves that your change is not likely due to chance and it represents a true effect or relationship between variables. The degree of dilution depends on how frequently users interact with the button and where measurement of the experiment is triggered. If the majority of users interact with the button, then the experiment will be less diluted, making it easier to reach statistical significance. However, if only a small percentage of users interact with the button, then the experiment will be more diluted, making it harder to reach statistical significance. To avoid dilution, product teams must carefully design their experiments as close to where the change is triggered, in this case, by starting to measure the experiment when a customer taps the button, and weigh up the potential impact of the user behaviour on the experiment's validity.
Before starting, have an idea of how long it will take to reach significance: As a growing company we have ambitious goals and want to deliver impact as quickly and safely as possible. In order to know that what you’re doing is right for customers it’s best to experiment. However, in order to achieve impact you need to be able to measure well and in a short time frame so that you can iterate and improve quickly. It’s important to understand how long your proposed experiment will take and weigh up if it’s worth experimenting at all. If you know upfront that it will take too long you can ask yourself the following questions:
If this is too small to measure, could you be working on more impactful changes instead?
If you have high confidence backed by research, first principles or similar previous experiments, do you need to run an experiment?
Is this a one-way or two-way door decision?
What are you trying to learn from the experiment - are there other ways you can learn?
It’s worth noting that you can do all of these things beforehand and only realise once you’ve started your experiment that it will take too long to reach significance. This was the case for some of our experiments, as the sample size took longer than expected or the change had less of a customer behaviour impact than we anticipated. In these cases we were able to disprove our hypothesis and use the learnings for our next experiments.
Focus on changes at the top of the funnel: Top of the funnel refers to the initial stage of the customer journey. Changes here allow you to affect the broadest set of customers, which will increase your potential impact. We noticed many customers were reporting fraud for transactions that should have been disputes. When we researched this problem we identified the root cause was that the language we used at the beginning of the journey was not clear enough, which led customers down the wrong route. We used this insight to make the language really clear and this helped thousands of customers get to the right flow and resulted in over £1m in savings each year.
Before:
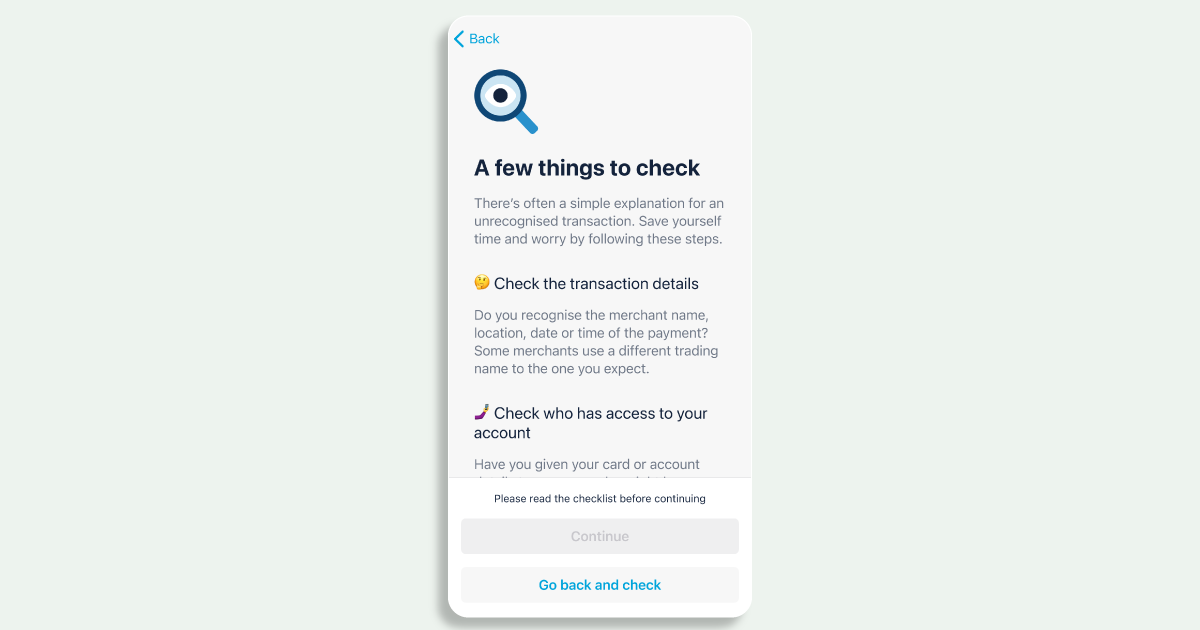
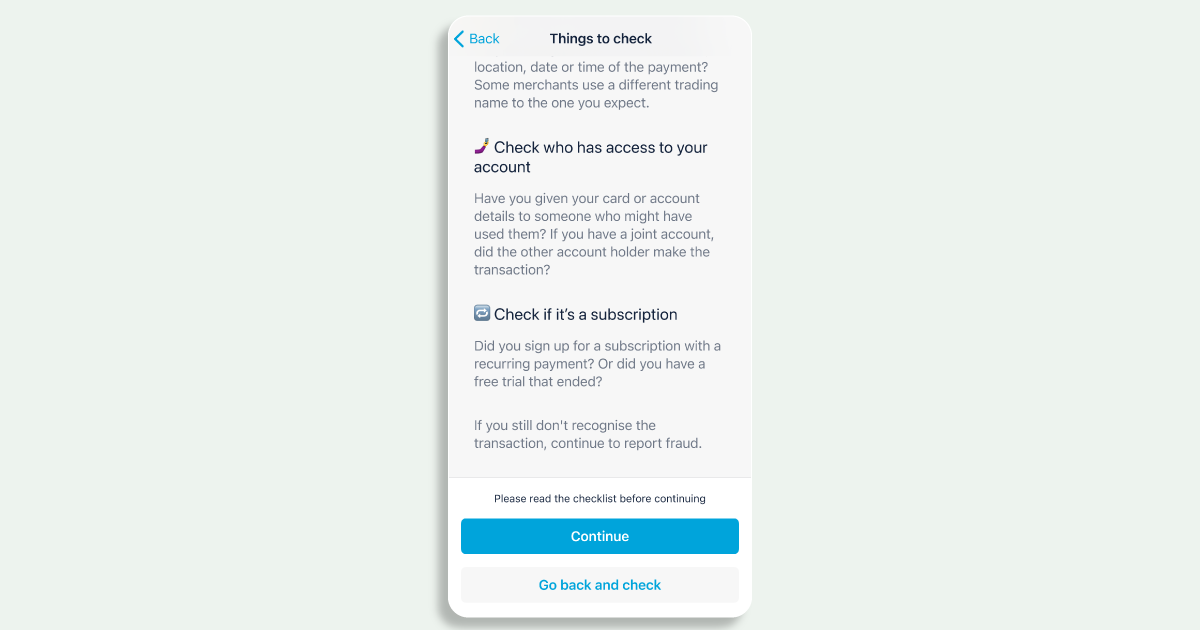
After:
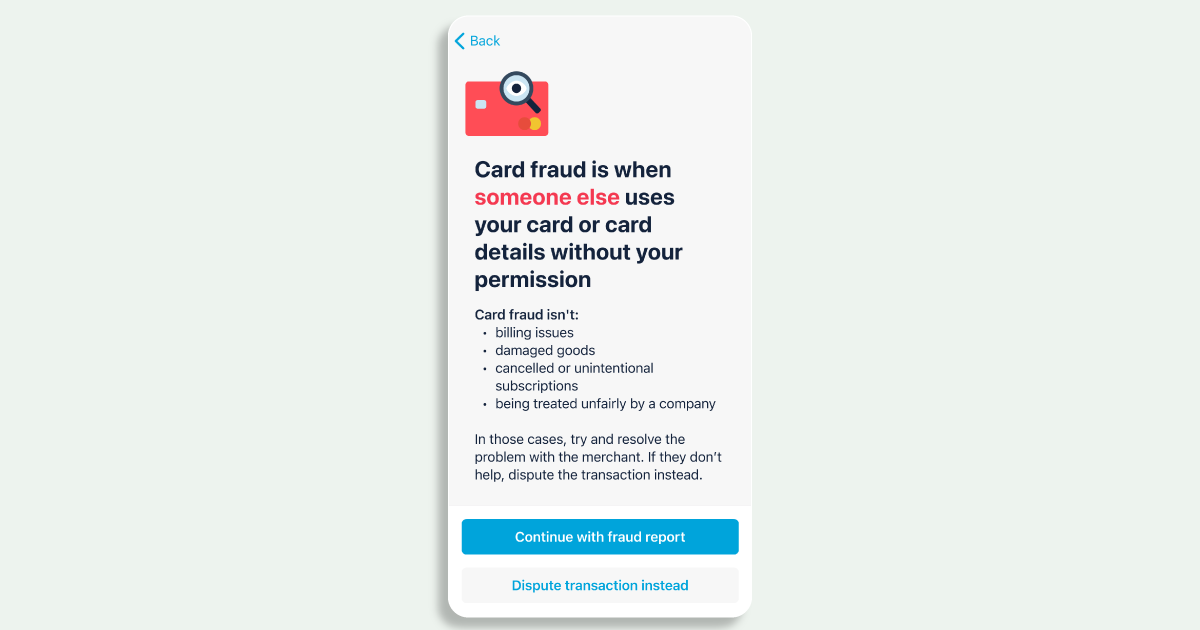
Fire Pellets, then Cannonballs
Our approach to experimentation has been pellets, then cannonballs - which Alex our Designer for Ops puts nicely in this blog post. The concept is to prioritise smaller effort experiments that have the ability to create high impact if they work well, and relatively low downside if they fail. Once you know you’re on the right track from a smaller experiment then put more effort towards it and do something bigger and bolder.
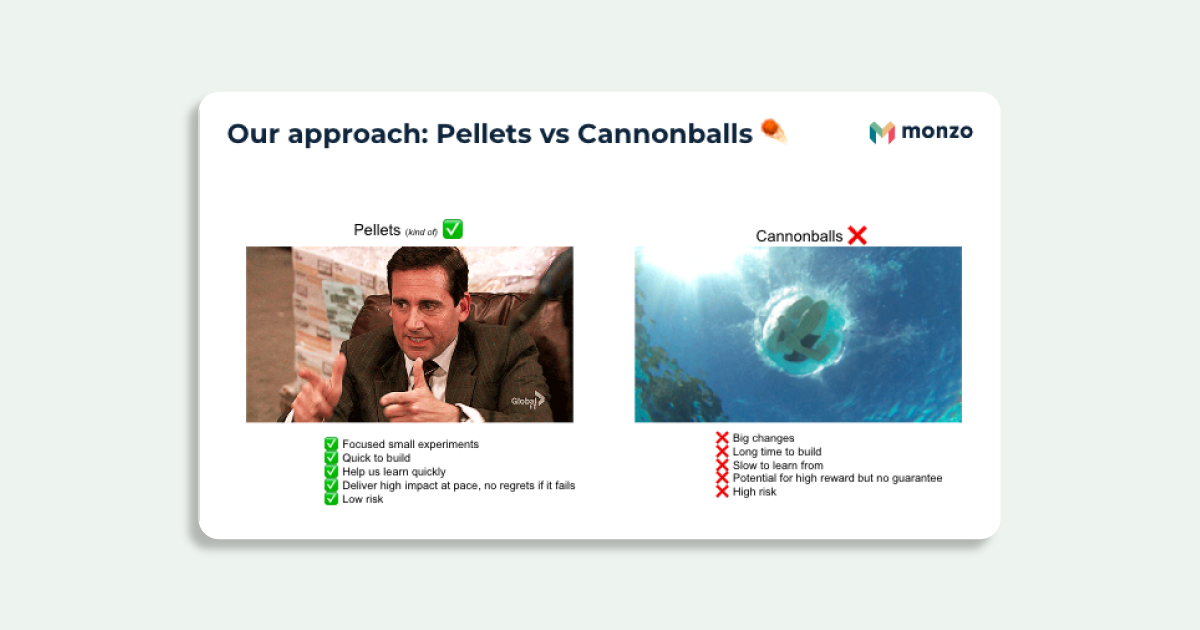
Investing in the tools and processes to move fast, has compounding gains
Monzo knows the importance of laying great foundations for data and two principles have guided the data team to enable a robust data stack:
Centralised data management - enabling a 360 degree view and alignment on how we all treat data
Decentralised value creation - having the data discipline embedded across the entire company
That makes running experiments easy and fast. We decided on our key metrics and guardrail metrics at the beginning of the half. This was great for easily spinning up new experiments and using the same metrics for each one made it easy for us to align on what was important. We’re able to easily double click into the data from a high level down to specific customer conversations. Data Scientists are embedded within the squad and are key when making decisions, they typically spend their time setting up experiments, writing experiment plans, impact sizing, analysing experiment results, determining future high impact work and more strategic work at a collective level.
In conclusion, experimenting is at the heart of Monzo's product development philosophy. Running experiments enables us to create better products and deliver effortless customer experiences. But, for us, it's not just about trial and error. We believe in having a clear hypothesis, measuring the right metrics, ensuring our experiments are well isolated, and having a well-defined timeline to achieve statistical significance.
The Customer Support Experience Squad has learned valuable lessons from the 43 experiments we launched in the past year. I’d like to thank the team and everyone who’s supported us to deliver so much for our customers.
As we continue to iterate and improve our product, we'd like to invite you to join the conversation. Do you have any valuable lessons from running experiments that you'd like to share? We'd love to hear about your experiences and how you approach running effective experiments. So, please share your thoughts and comments below.
Think you'd like to work on this stuff at Monzo? Check out our open roles below: